GPT-4 vs Claude vs Gemini: Choosing the Best LLM for Your Content Strategy
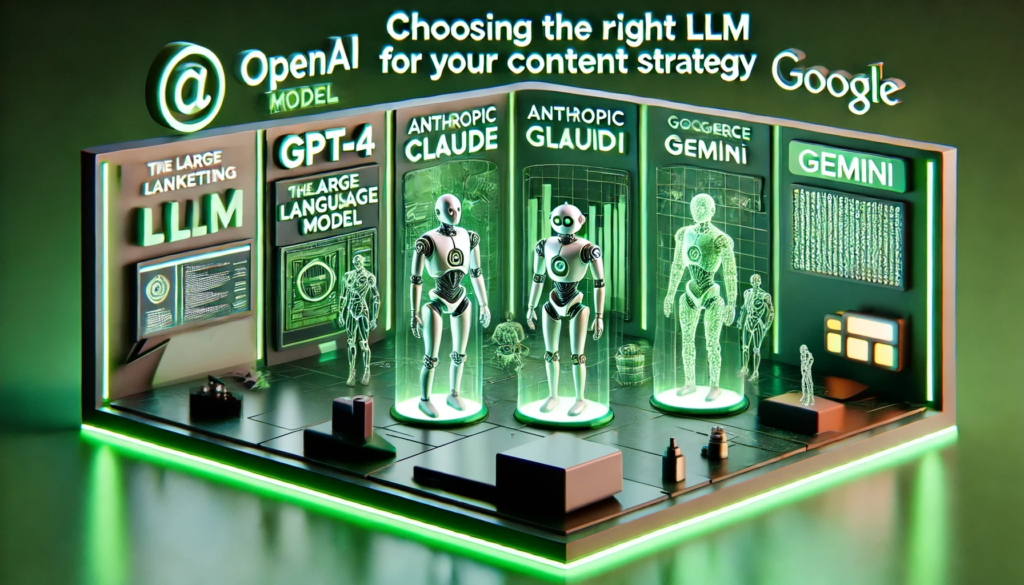
Choosing the right large language model (LLM) can make or break your content strategy. Whether you’re running a startup, managing an e-commerce platform, or spearheading campaigns in a digital marketing agency, the ability to produce high-quality, engaging content quickly is paramount. This is where advanced LLMs come into play, transforming your content creation process by leveraging the power of AI.
At Dapta, we understand the unique needs of businesses striving to maintain creativity while optimizing their marketing efforts. Our mission is to democratize AI, empowering everyone to simplify automation and enhance operational efficiency. In this blog post, we’ll explore the top contenders in the LLM space: OpenAI’s GPT-4, Anthropic’s Claude, and Google’s Gemini. Each of these models offers unique strengths and capabilities, but which one is the best fit for your content needs?
We’ll delve into the specifics of each model, comparing their performance, versatility, and ease of integration. By the end of this article, you’ll have a clear understanding of which LLM can best help you unlock your content’s potential, ensuring your marketing campaigns remain innovative and effective.
Understanding Large Language Models (LLMs)
Large language models (LLMs) are advanced AI systems trained on vast amounts of data to understand and generate human-like text. These models, such as GPT-4, Claude, and Gemini, have revolutionized content creation by enabling users to produce high-quality text quickly and efficiently.
LLMs are designed to process and generate text that closely mimics human language. They achieve this by leveraging complex neural networks, particularly transformer architectures, which allow them to understand context, semantics, and syntax at an unprecedented scale.
What are Large Language Models?
Large language models are AI systems that use deep learning techniques to process and generate text. They are trained on extensive datasets comprising diverse text sources, including books, articles, and websites. The primary goal of LLMs is to understand and generate text that is coherent, contextually relevant, and human-like.
For instance, OpenAI’s GPT-4, one of the most well-known LLMs, contains billions of parameters that enable it to perform a wide range of language tasks, from translation to content creation. Similarly, Anthropic’s Claude and Google’s Gemini are notable examples of LLMs that excel in various applications.
How Do LLMs Work?
The working mechanism of LLMs involves several stages, primarily centered around the transformer architecture. Transformers use an encoder-decoder structure with self-attention mechanisms to process text. Here’s a simplified breakdown:
- Data Collection: LLMs are trained on vast datasets that include text from various sources like books, articles, and websites.
- Preprocessing: The text data is preprocessed into tokens, which are numerical representations of words or subwords.
- Training: The model learns to predict the next token in a sequence, adjusting its parameters to minimize prediction errors. This process involves billions of parameters and requires significant computational resources.
- Fine-Tuning: After initial training, LLMs can be fine-tuned on specific datasets to improve performance on particular tasks.
Statistics highlight the scale and capabilities of these models. For example, GPT-4 has 175 billion parameters, making it one of the largest and most powerful LLMs available.
Significance of LLMs in Modern Digital Landscape
The impact of LLMs on the digital landscape is profound. They have transformed how businesses approach content creation, customer service, and data analysis. Here are some key areas where LLMs make a significant difference:
- Content Creation: LLMs enable the generation of high-quality, engaging content quickly, which is invaluable for marketing and digital campaigns.
- Customer Service: AI-powered chatbots and virtual assistants, driven by LLMs, provide efficient and accurate customer support.
- Data Analysis: LLMs assist in processing and analyzing large volumes of text data, uncovering insights and trends that inform business strategies.
Comparing Top LLMs: GPT-4, Claude, and Gemini
In the competitive landscape of large language models (LLMs), three names stand out: GPT-4 by OpenAI, Claude by Anthropic, and Gemini by Google. Each of these models brings unique strengths and capabilities to the table, making them suitable for different content creation needs.
GPT-4
GPT-4 is the latest iteration in OpenAI’s series of generative language models. Known for its large parameter count and impressive performance across various tasks, GPT-4 has set a high standard in the AI community.
Strengths:
- Excels in generating coherent and contextually relevant text
- Particularly effective in creative writing, summarization, and translation tasks
- Ability to handle complex prompts and produce high-quality outputs
Weaknesses:
- Can sometimes produce outputs that are overly verbose or lack factual accuracy
- High computational requirements can be a barrier for some users
Unique Features:
- Extensive training on diverse datasets allows it to understand and generate text in multiple languages
Real-world Application: A digital marketing agency used GPT-4 to generate personalized email campaigns for different customer segments. The model’s ability to understand context and brand voice resulted in a 25% increase in email open rates and a 15% boost in click-through rates.
Integration Process:
- Obtaining API access from OpenAI
- Setting up authentication
- Sending requests to the API with your prompts
- Processing and implementing the responses in your workflow
Challenges may include managing API rate limits and costs, as well as fine-tuning prompts for optimal results
Claude
Strengths:
- Robust safety mechanisms that reduce the likelihood of generating harmful or biased content
- Performs well in tasks that require ethical considerations and sensitivity
Weaknesses:
- Focus on safety can sometimes limit the model’s creativity and flexibility
- May be less versatile compared to GPT-4
Unique Features:
- Incorporates advanced safety protocols and ethical guidelines
Real-world Application: A healthcare company utilized Claude to generate patient educational materials. The model’s focus on accuracy and ethical considerations ensured that the content was not only informative but also adhered to strict medical guidelines, resulting in improved patient understanding and compliance.
Integration Process: Integrating Claude typically involves:
- Applying for access through Anthropic
- Setting up API credentials
- Implementing Claude’s API in your application
- Crafting prompts that align with Claude’s ethical guidelines
The main challenge is often adapting to Claude’s more constrained outputs, which may require refining prompts to achieve desired results while maintaining ethical standards.
Gemini
Gemini, Google’s entry into the LLM space, combines extensive data resources with cutting-edge AI research. This model is designed to integrate seamlessly with Google’s ecosystem of tools and services.
Strengths:
- Integration with Google’s suite of products enhances its utility for users already embedded in the Google ecosystem
- Excels in tasks related to data analysis and natural language understanding
Weaknesses:
- Performance can be inconsistent across different types of tasks
- Reliance on Google’s infrastructure may pose challenges for users seeking independent solutions
Unique Features:
- Ability to leverage Google’s vast data resources and AI advancements
Real-world Application: An e-commerce platform integrated Gemini into their product description generation process. By leveraging Gemini’s access to Google’s vast product knowledge, they were able to create more accurate and SEO-friendly product descriptions, leading to a 20% increase in organic search traffic.
Integration Process: Integrating Gemini typically involves:
- Setting up a Google Cloud account
- Enabling the necessary APIs
- Implementing Gemini through Google’s AI platform
- Configuring the model to work with your existing Google services
The main challenge is often navigating Google’s complex ecosystem and ensuring proper data handling practices to comply with Google’s policies.
Ethical Considerations and Limitations of Using LLMs for Content Creation
While LLMs offer immense potential for content creation, it’s crucial to consider the ethical implications and limitations of these powerful tools:
- Bias and Fairness: LLMs can inadvertently perpetuate biases present in their training data. Content creators must be vigilant in reviewing and editing AI-generated content to ensure it’s fair and inclusive.
- Misinformation: LLMs can sometimes generate plausible-sounding but incorrect information. Fact-checking AI-generated content is essential, especially for sensitive topics.
- Copyright and Originality: There are ongoing debates about the copyright status of AI-generated content. Ensure you understand the legal implications of using LLM-generated material in your content strategy.
- Over-reliance on AI: While LLMs are powerful tools, they should complement human creativity rather than replace it. Maintain a balance between AI-generated content and human insight.
- Privacy Concerns: Be mindful of how you use and store data when working with LLMs, especially when dealing with sensitive or personal information.
- Transparency: Consider disclosing when content is AI-generated or AI-assisted, particularly in contexts where authenticity is crucial.
Choosing the Right LLM for Your Needs
Selecting the right large language model (LLM) depends on your specific content creation needs and goals. Consider the following factors:
- Performance: Evaluate each model’s accuracy, speed, and scalability about your content requirements.
- Cost: Consider subscription fees, computational resources needed, and ongoing maintenance costs.
- Ease of Use: Assess how easily the model can be integrated into your existing systems and workflows.
- Specific Use Cases: Match the model’s strengths with your particular content needs, whether it’s creative writing, data analysis, or ethically sensitive content.
- Ethical Alignment: Choose a model that aligns with your organization’s ethical standards and content guidelines.
The Future of Content Creation with LLMs
As we have explored, selecting the right large language model (LLM) is crucial for optimizing your content strategy. Each model—GPT-4, Claude, and Gemini—brings unique strengths to the table, catering to different needs and preferences.
Incorporating LLMs into your content strategy can revolutionize your approach to marketing, customer service, and data analysis. These models enable rapid, high-quality content creation that can keep your campaigns innovative and engaging. By leveraging the power of LLMs, businesses can maintain a competitive edge in the digital landscape.
Looking ahead, the advancements in LLM technology promise even greater capabilities and applications. From enhanced accuracy and reduced bias to new possibilities in multimodal content creation, the future of LLMs is bright. As these models continue to evolve, their impact on various industries will only expand, offering more sophisticated tools for content creation and beyond.
To explore how you can leverage these powerful LLMs in your content strategy, create your account with Dapta today and start transforming your approach to AI-powered content creation.